Will a new song resonate with an audience? Is it marketable? What are its chances of becoming a success?
These questions linger over artists, managers, and record labels of every size. It makes careers in music and drives the industry. And yet, the question was much harder to answer until very recently. Sure, some folks legendary in music history have had an exceptional ear, like Clive Davis, skilled at picking out generational talents. Today, the answers to these all-important questions are possible and accessible.
In fact, it recently becomes possible for anybody to find answers in just a few clicks, whether you, me, or someone new to the industry. With the right software, the answer is never more than an audio file and three minutes away.
Teaching Machines to Understand Music
Using machine learning and a library of songs, Unbias can provide the answer. It does so by teaching artificial intelligence using this bank of songs and then inviting it to listen to the song under evaluation. After a few minutes — which the AI uses to sweep thousands of songs — it can predict with striking accuracy whether an unreleased song will become a success.
A common approach to evaluate the accuracy of an AI is to compare it to human-level error. We calculate how often we get things wrong at specific tasks, which is known as an error rate. For example, if you listen to 10 random songs that were released in the past 90 days and the artists are unfamiliar to you — then you pose the question, “which of the 10 songs generated most listens?”
- A typical listener might get 6/10 correct, which equates to a 4% error rate.
- A typical A&R professional might get 8/10 correct, 2% error rate.
- You bring in Clive Davis; he might get 9/10 correct, 1% error rate.
- You bring in clones of Clive Davis’s; they might get 9.7/10 correct, 0.3% error rate.
So in this example, how would you define the human-level error? Is it 4%, 2%, 1% or 0.3%?
If you picked 0.3%, then you are correct, a team of experts equates to minimal human-level error. In data science, that theoretical minimal limit is called Bayes Error. This means that at some point, a human being will be so accurate that no one can’t surpass that level. Low error rates are good.
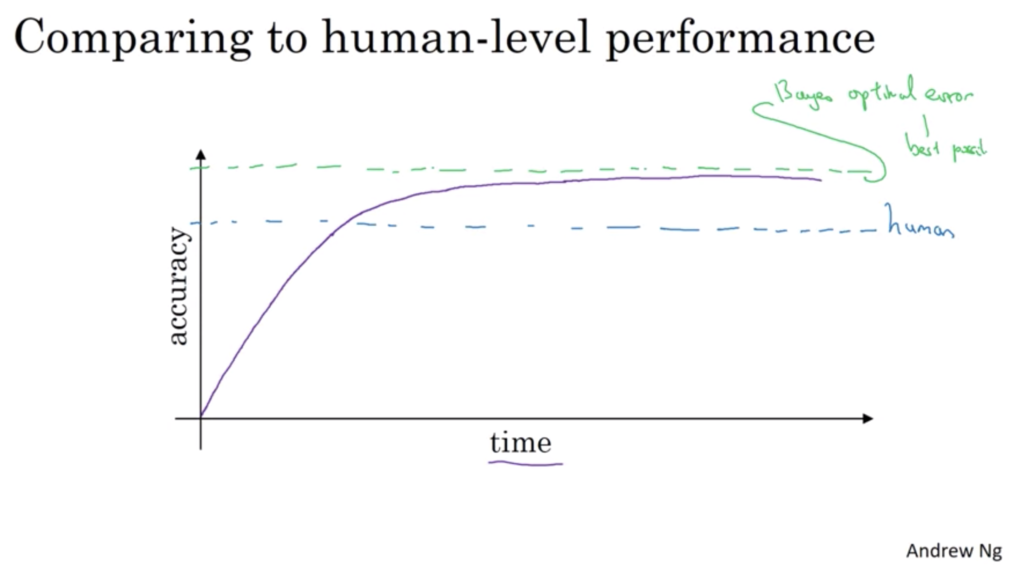
The point is that AI, when trained with enough data, can outperform humans at some tasks. Think about chess or the ancient Chinese game Go. There are human grandmasters in both, experts who have achieved an incredible ability. And yet with advanced machine learning, computers can beat the best humans.
Some tasks are more stringent than others. For example, picking stocks or a combination of winning lottery numbers is still very hard to do for humans. Therefore it will be very hard for an AI.
The same is true for predicting a song’s success, which is probable with experience and time. With the right software and AI, you can bring a grandmaster-beating capability to your fingertips. The goal isn’t to put A&R experts out of a job or industry professionals in general, but to become more effective in your decisions and increase your chances of success with Unbias.
Today, most of the music industry collects data from verticals like social media, playlists, streams, YouTube comments and try to make predictions about how the artist is going to perform. They stopped listening to the music. While they are great at marketing music, their mistakes of identifying and modifying potential projects are becoming costly and based more on gut instincts than raw data. Our approach is another accurate way to find out or fine-tune your personal evaluation of a song. We’re able to predict within 88% accuracy how well a piece is going to perform.
The distinction here is not to answer if the music is good or bad, but if it is high-performing or not based on today’s consumer listening habits. There are obvious indicators that don’t need prediction, especially in dominating categories like Hip-Hop, Pop, and Dance, but that’s only half of the answer. Explaining the rise of specific sub-genres or the re-emergence of old classics is hard to do, and attributing it to luck or virality, in my opinion, is lazy thinking.
The back of every reality is a cause. Often the reason is so far removed from the effect that the circumstance can be explained only by attributing it to luck.
I believe everything having a real existence is capable of proof, and using Unbias is part of the answer.
Unbias’s Roots in Music and Tech
Originally from Sudan, I came to the U.S. to study computer science in college, and started in the music business on the broadcasting side at SiriusXM in 2005. Soon, I started to dabble in music production. Then I began DJing locally for fun in Washington D.C. while working at SiriusXM.
In 2012, I left SiriusXM for a product idea to help artists acquire more bookings. It was a marketplace for uniting artists and venues. Scaling two-sided marketplaces was demanding and challenging—so I moved on after a year. Then I went to L.A., where I was CTO of a music streaming company. Next, I launched a startup that used machine learning to make political predictions. I learned campaigns plan their media spend on how many voters they can engage, a useful lesson applicable to the music industry. After politics, we even had a short six-month stint modeling healthcare data in Detroit.
Now, as I look back, I see all the places where I could have improved, and can translate all the learned experiences across different industries. Ten years later, it’s with a newfound perspective that I return to the world of music.
Designing an AI with a Golden Ear
Unbias really began with the creation of an AI. In the beginning, I asked myself, “If an AI wanted to evaluate music, how would it do it?”
Incorporating data scientists into the early development, I began from a simple premise: We would want the AI to look at the music similar to how an AI looks at pictures and pixels. In image recognition tasks, AI looks at details down to each pixel and shades of gray and colors, and they can learn, pixel by pixel, then group pixels to understand patterns — this is a bird, this is a dog. If we borrow that approach, we can actually do something like that in music.
Unbias AI turns audio files into a spectrogram, a heatmap of a song’s frequency over time. The machine-learning model takes “snapshots” every second of the spectrogram, letting the AI record data points on the countless components that make up the frequency spectrum, including genre, instruments, mood, and so on. Once the AI understands a song, it starts finding patterns in others.
The AI runs comparisons against thousands of songs to learn even more about the unreleased song and where it fits into the broader picture in today’s music industry. How does the AI know what songs to compare against? The exact formula is one of Unbias’s secret ingredients. Still, essentially we use a representative sample of songs that speak to exactly what’s performing well in the music industry right now, across most genres.
The AI then spits out its final prediction. It even charts how it expects songs to perform in the time after release. At first, our team predicted with 50 percent accuracy, then 60 percent, they kept incorporating data and refining our AI until it reached the 88 percent where it stands today.
How Can Labels Use Unbias to Do Better Business?
Unbias can predict a song’s chances of success. But from here, another question remains: how can labels use this prediction? In at least 10 ways.
- The AI’s prediction can be one valuable piece of information used in a label’s decision about whether to sign a new artist. With hard data, labels can feel better about which artists are the best to bring on board.
- An artist is about to release a single. Songs have been recorded. But which one should be released as the single? The AI’s prediction can help make the call. In the same way, an artist cutting an album can use the AI to select final tracks for the album, and to know which of its tracks to promote the most.
- It’s time to spend some media dollars. Which artists and tracks deserve bigger pieces of the media budget pie? In predicting which songs, features, playlists, and artists will perform well, Unbias can help labels know what to promote and to what audience.
- Unbias can help with a difficult task — selecting what tracks to leave behind, a potentially sensitive subject. If the AI has low confidence in a track, it makes sense to leave that one on the cutting room floor.
- Which artists should be placed together? When combing through artist catalogs, the AI can detect similarities in musical features, genres, and playlists, helping labels know which artist pairings might make a synergistic fit for cross-promotion.
- After a song has been released, Unbias can track its progress against benchmarks. It gives a data-driven picture of how a song performs over time.
- It might sound strange, but the AI can identify moods and feelings. This can help labels make decisions about licensing music to commercials or films.
- Most artists have a trove of unreleased music. Unbias can help labels determine which tracks might be worthy of digging up for release.
- The Unbias AI can help labels identify promising target playlists for new tracks. Knowing for sure what playlists are the best fit for your music can help you target the right playlists from the beginning, saving time and money.
- A good product always finds its way to market. At the end of the day, Unbias can tell you if you have a good product.
Getting Started with Unbias
To get started, simply create an account on Unbias. You get three free songs to begin. The way it works is you upload an audio file and fill out metadata (like song name, genre, and whether the song has been released). From there, the AI delivers results in about three minutes.
Since we launched Unbias in October 2020, our team have worked diligently to refine the AI even further. They are still honing the AI today, improving its ability to see deeply into every last second of music. We release a new model every month, maybe more frequently. And with time, the listening ability and golden “ear” of the AI — already at the level of a master — will only get better.